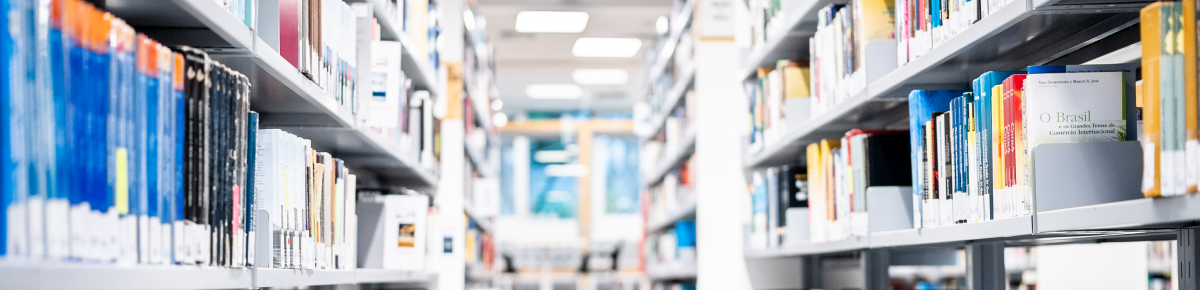
Destaques
Comunidades no Repositório
Selecione uma comunidade para navegar por suas coleções
Submissões Recentes
Emendas reelegem um prefeito?: Estudo quantitativo sobre o impacto das emendas parlamentares nas eleições municipais
(2025) Casais, Bianca; Wang, Henrique
Caderno de Estudos: Melhores Reportagens de Análise Contábil 2025.1
(2025) Camila Boscov (Orientadora); CAMILA PEREIRA BOSCOV; Alva, Marina Moretti (Org.); Casarotti, Maria Eduarda (Org.)
Este caderno, elaborado pelos voluntários do Blog de Cont, reúne as melhores reportagens de análise contábil produzidas pelos alunos do 2º semestre dos cursos de Administração e Economia (2025.1) do Insper, na disciplina de Contabilidade Financeira, sob orientação da professora Camila Boscov e do professor-monitor Fabio Henrique de Souza.
As aulas em sala foram conduzidas pelos professores: Camila Boscov, responsável pelas turmas A e B; Cristiane Kusaba, pela turma C; e Marcelo Ermel, pela turma D. Os processos de avaliação e correção dos trabalhos produzidos pelos estudantes contaram com a dedicação do professor Fábio Henrique de Souza, nas turmas A e B, e da professora Ivanice Teles, nas turmas C e D.
O material reflete o compromisso dos alunos com a excelência e a aplicação prática dos conceitos aprendidos.
Inclusão Produtiva: eficácia dos programas sobre emprego e renda
(2025) Pignatari Filho, Carlos Eduardo
A pobreza não é um problema passageiro e afeta gerações de comunidades em vários países. Após
a pandemia de SARS-CoV-2 em 2019, o cenário de pobreza se agravou em uma crise sanitária e
econômica. No Brasil, foi ainda mais severa, como mostram os indicadores socioeconômicos. É
crucial reconhecer que o sofrimento foi vivenciado de forma desigual, com mulheres, jovens e
pessoas negras sendo desproporcionalmente mais afetadas. Durante as crises, políticas de
transferência de renda desempenharam um papel de oferecer alívio imediato às populações mais
vulneráveis, evitando o agravamento da pobreza. Contudo, essa natureza emergencial não resolveu
problemas estruturais. No contexto pós-pandemia, a responsabilidade de erradicar a pobreza recaiu
sobre programas de inclusão produtiva que ainda não possuem os efeitos comprovados. Esse
trabalho busca responder qual a eficácia do impacto de um programa de inclusão produtiva no
longo prazo com base em uma resenha das meta-análises e revisões sistemáticas de literatura
mundial. Dito isto, encontrou-se evidências de que programas de treinamento apresentam efeitos
positivos em empregabilidade e vão aumentando ao longo do tempo. Assim como há evidência de
que transferência de renda não atrapalha em ocupação e rendimento nos países em
desenvolvimento. Por fim, há efeitos contraditórios sobre empregabilidade para programas de
intermediação de mão de obra e, inclusive, do comportamento ao longo do tempo.
Ascensão, permanência e influência: O caso das líderes mulheres no Governo Federal Brasileiro.
(2025) Carvalho, Marcela Garcia
Nas últimas décadas, a participação feminina no mercado de trabalho e no setor público tem crescido, embora barreiras estruturais ainda limitem o acesso de mulheres, especialmente negras, aos cargos de alto escalão. No Executivo Federal brasileiro, apesar do aumento geral da presença feminina desde a redemocratização, essa tendência não se reproduz nos altos escalões. A interseccionalidade de gênero e raça agrava a sub-representação de mulheres negras, pardas e indígenas. A literatura sobre burocracia representativa destaca a importância de uma administração pública cuja composição reflita a diversidade da sociedade, porém se concentram majoritariamente nos burocratas de nível de rua. Já a literatura sobre lideranças, apresenta um potencial efeito cascata (trickle-down) da diversidade nos níveis mais altos de uma organização, nos demais níveis. Desse modo, a partir de dados disponibilizados pela Escola Nacional de Administração Pública (ENAP) e utilizando Modelos de Sobrevivência, Regressões Quantílicas, Logarítmicas e Múltiplas, este estudo analisa as dinâmicas de ascensão, permanência e influência de mulheres brancas e negras nos cargos alto escalão do Executivo Federal entre 2000 e 2023, avaliando se (i) mulheres, especialmente mulheres negras, têm menor probabilidade de chegar aos cargos de alto escalão; (ii) se elas demoram mais que os homens para alcançar essas posições; (iii) se elas permanecem nessas posições menos tempo que os homens; e (iv) se, ao alcançarem o alto escalão, são mais prováveis de promover a ascensão de outras mulheres. Os resultados sugerem que mulheres têm menor probabilidade de ascensão aos cargos do alto escalão, sendo as mulheres negras as mais afetadas, embora não haja diferenças estatisticamente significativas no tempo de promoção ou permanência entre as que alcançam o topo. Ainda, observou-se um efeito cascata positivo, sugerindo que lideranças femininas e negras contribuem para uma burocracia mais representativa.